Discover our research, insights, and client successes to help you navigate change and accelerate value creation
Featured Insights
How Is Manufacturing on Data-Rich Platforms Different?

Featured Insights
Press Release
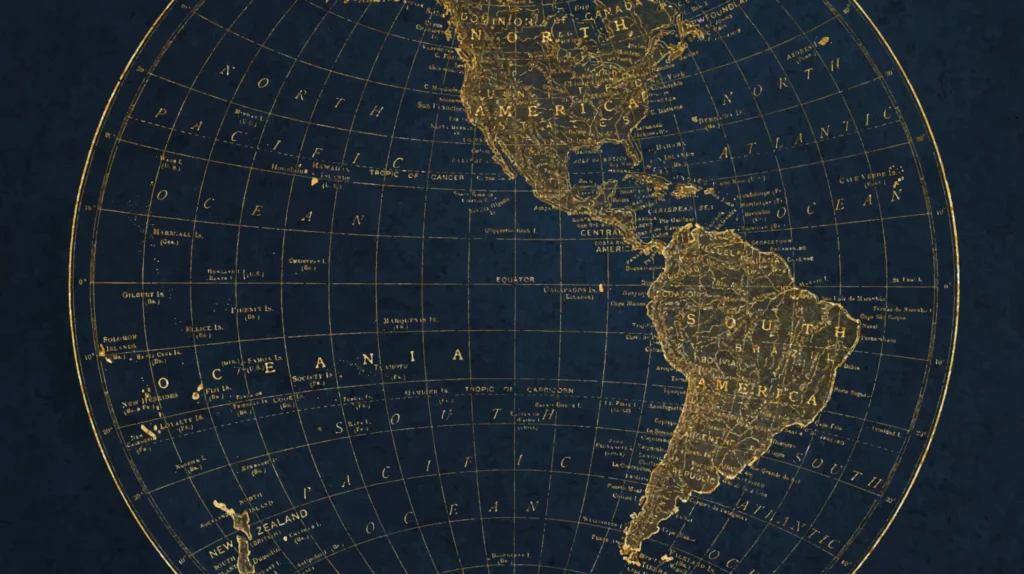
Featured Insights
How to Generate Value with Data Monetization in Telecom
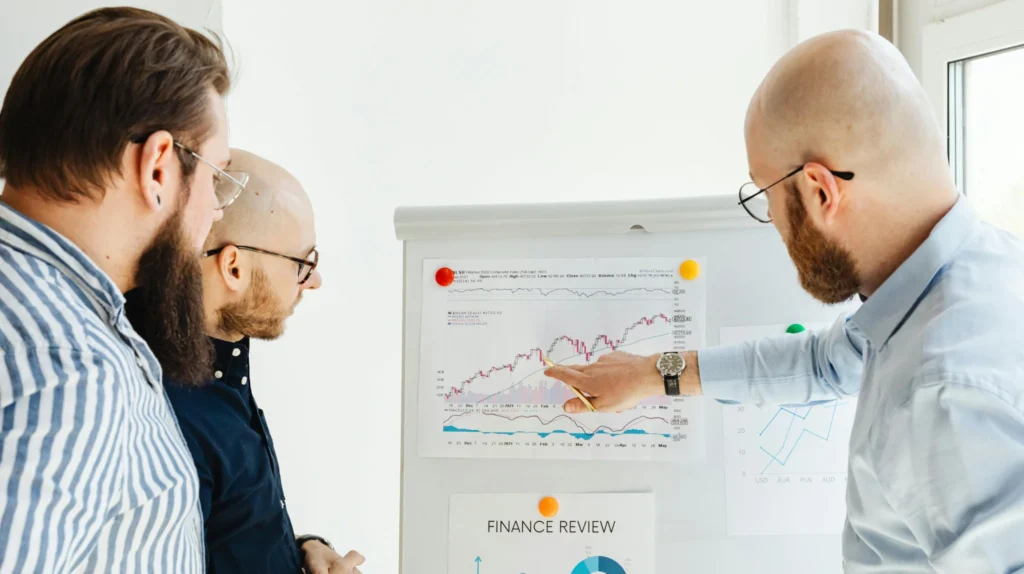
Featured Insights
The Evolution of Quality Assurance: How AI is Changing the Testing Game
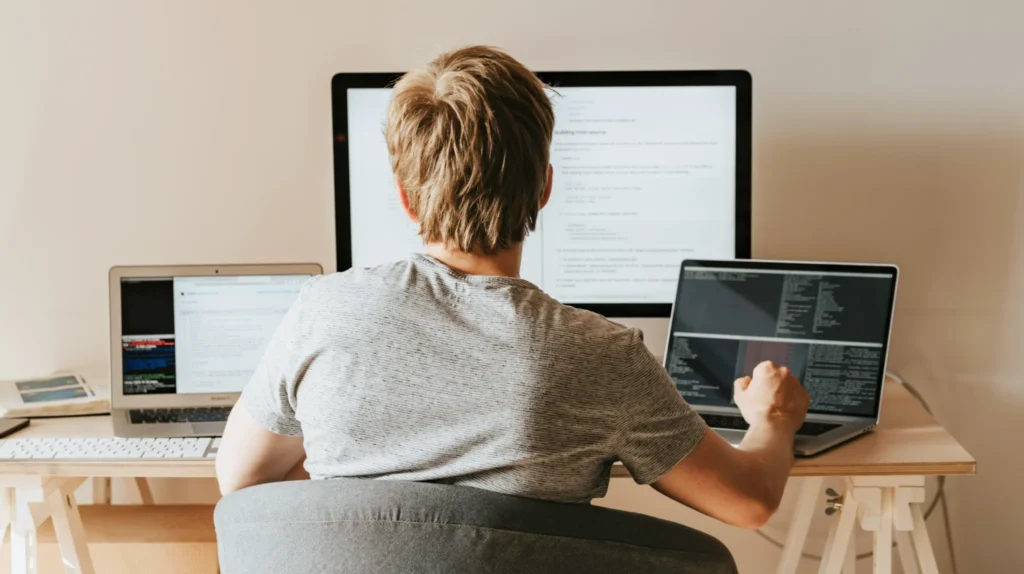
Featured Insights
The Importance of Efficient Data Governance in Clinical Trials
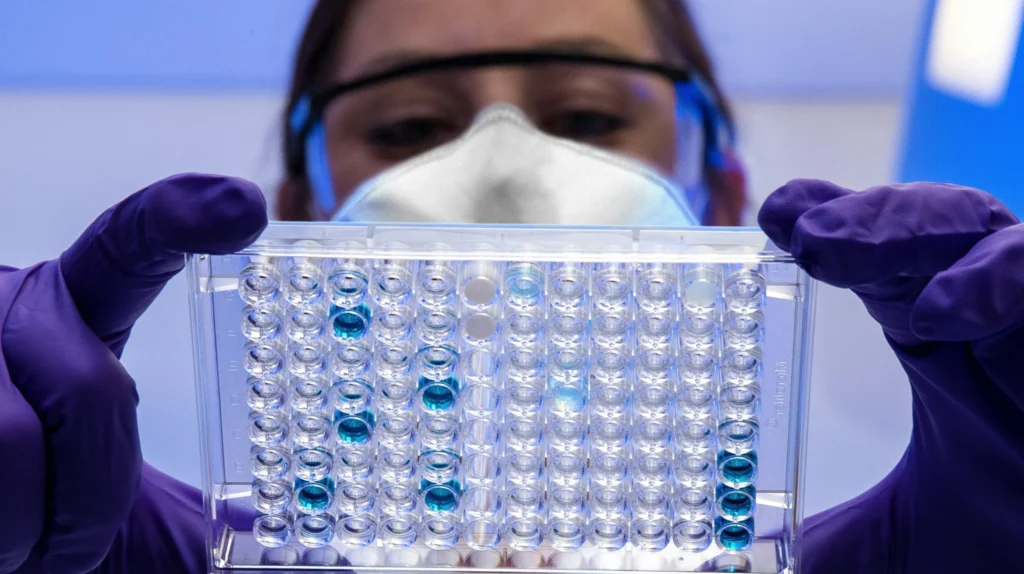
Featured Insights
Press Release
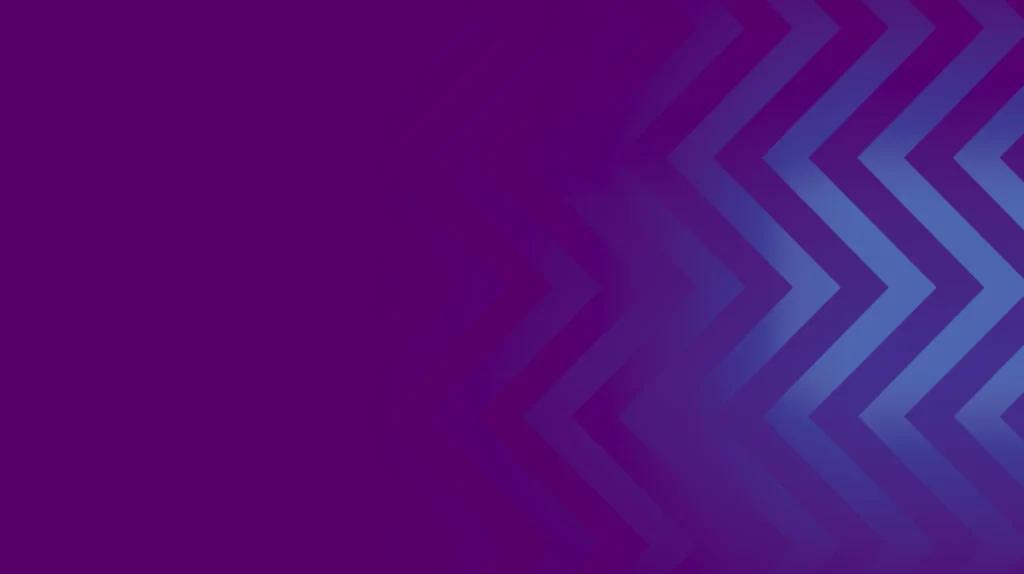
Featured Insights
The Future of OSS/BSS in the Modern Telco Space
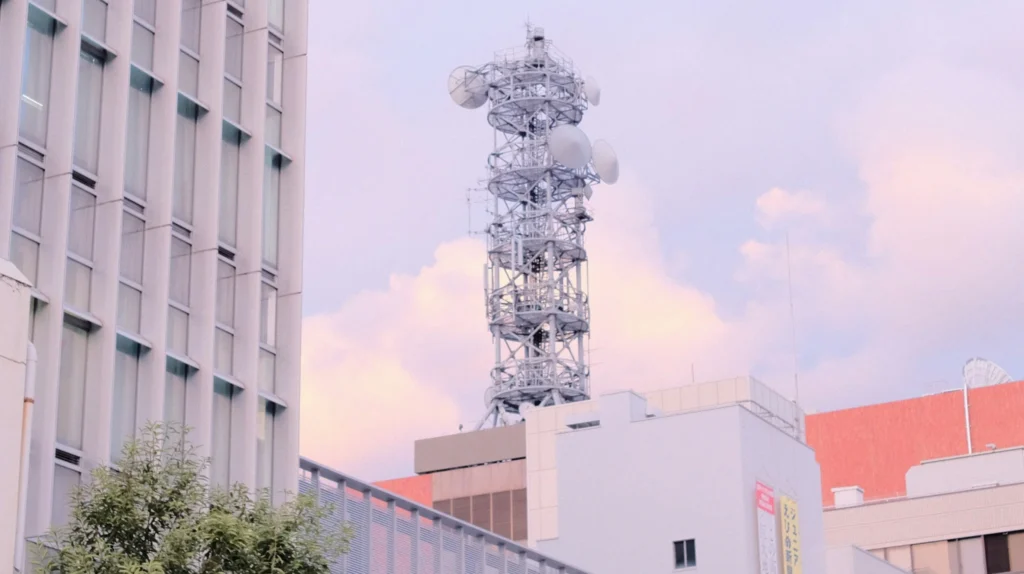
Featured Insights
Press release
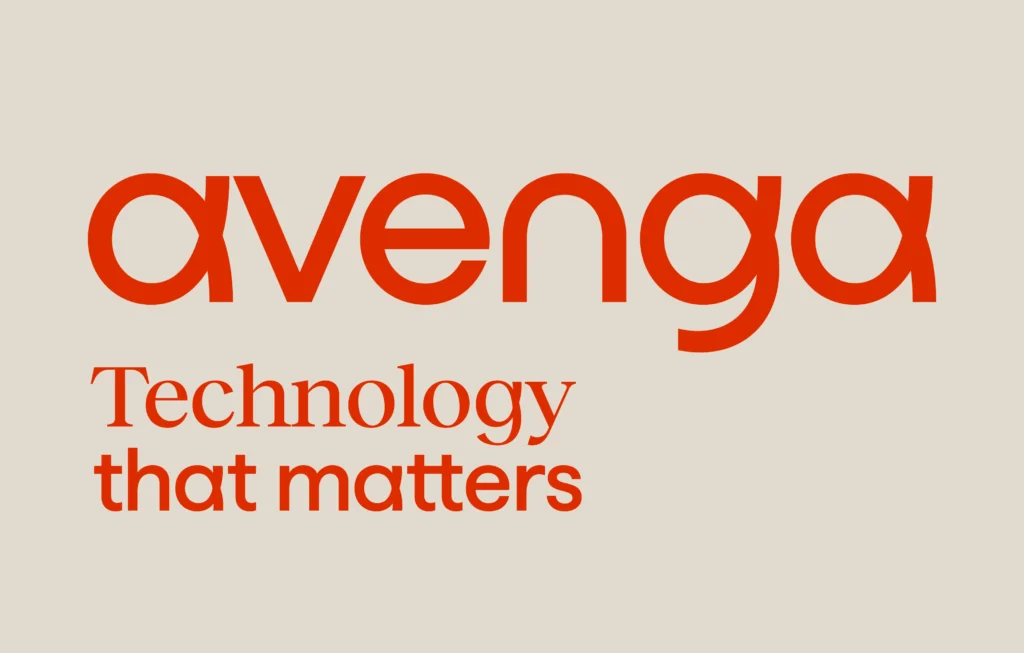
Featured Insights
Microsoft Power Automate: How To Use Cloud Flows for Process Automation
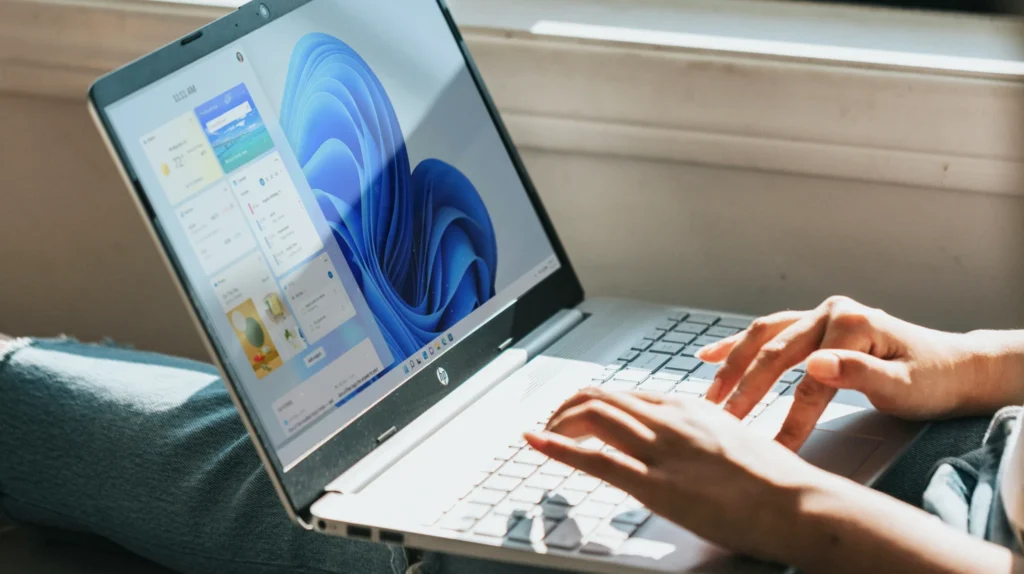
Featured Insights
What Is Azure Key Vault? A Beginner’s Guide and Use Cases
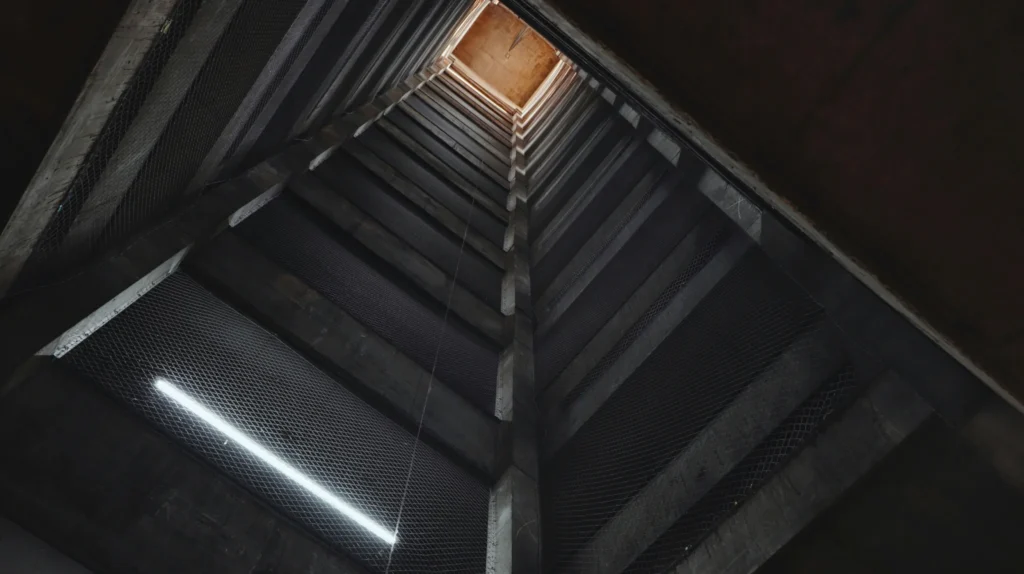
Featured Insights
Generic API or Back-End for Front-End? You Can Have Both
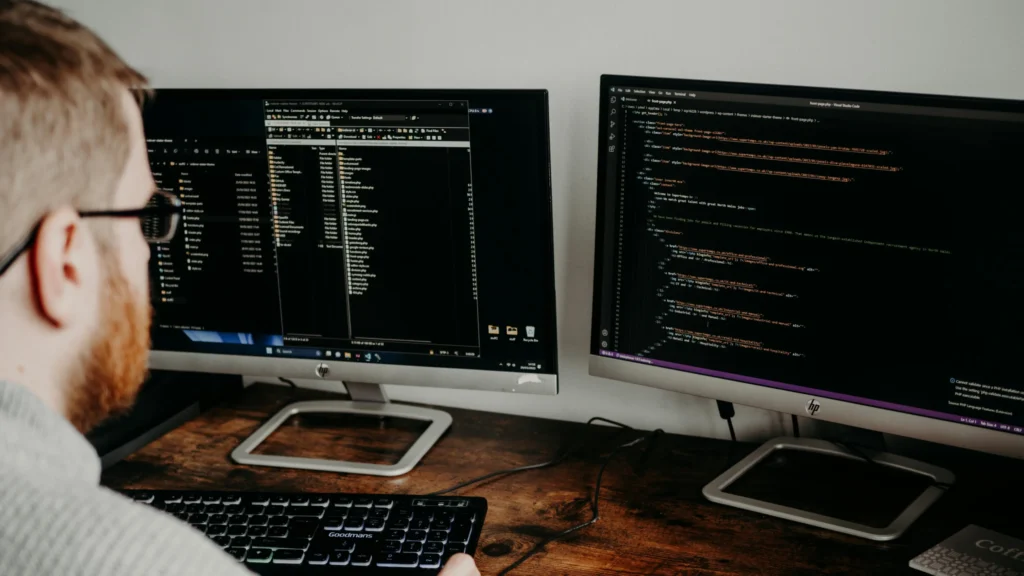
Featured Insights
Machine Learning Research Problems
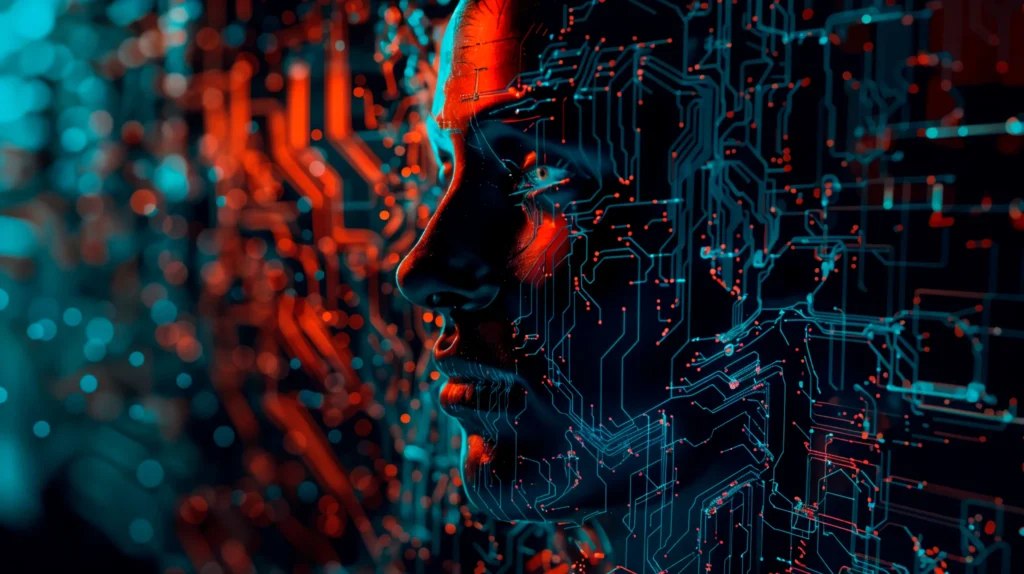
Featured Insights
Satellite Technology In 2025 And Beyond: The Future of GEO – Avenga
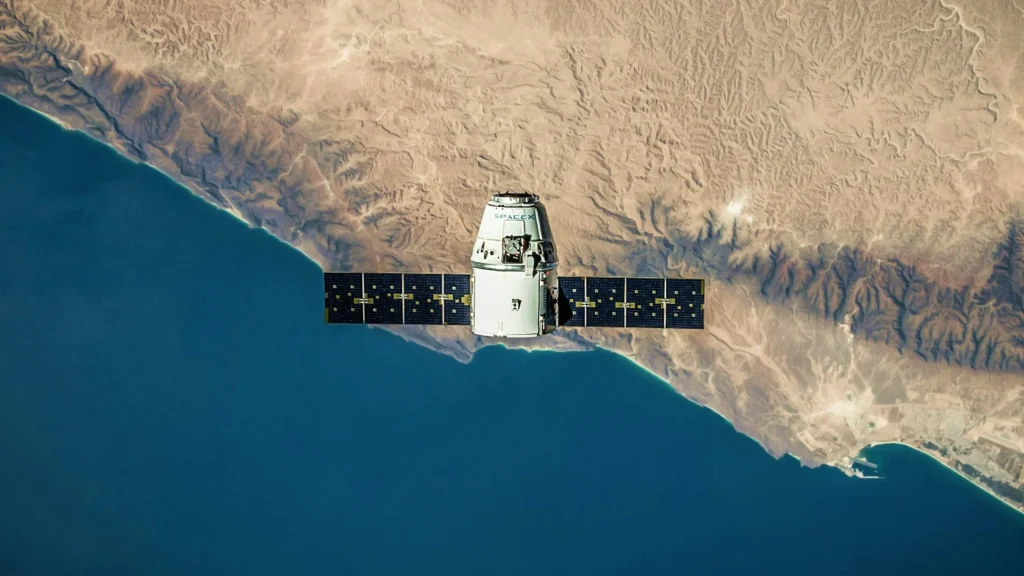
Featured Insights
Manufacturing Trends to Run 2025: A Practical Overview
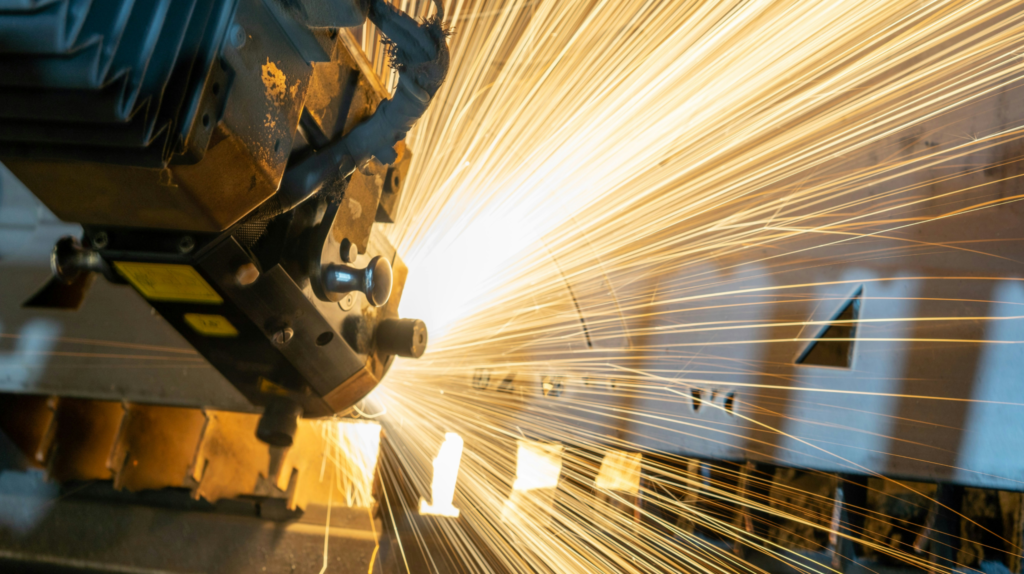
Featured Insights
Machine Learning Vs Traditional Programming
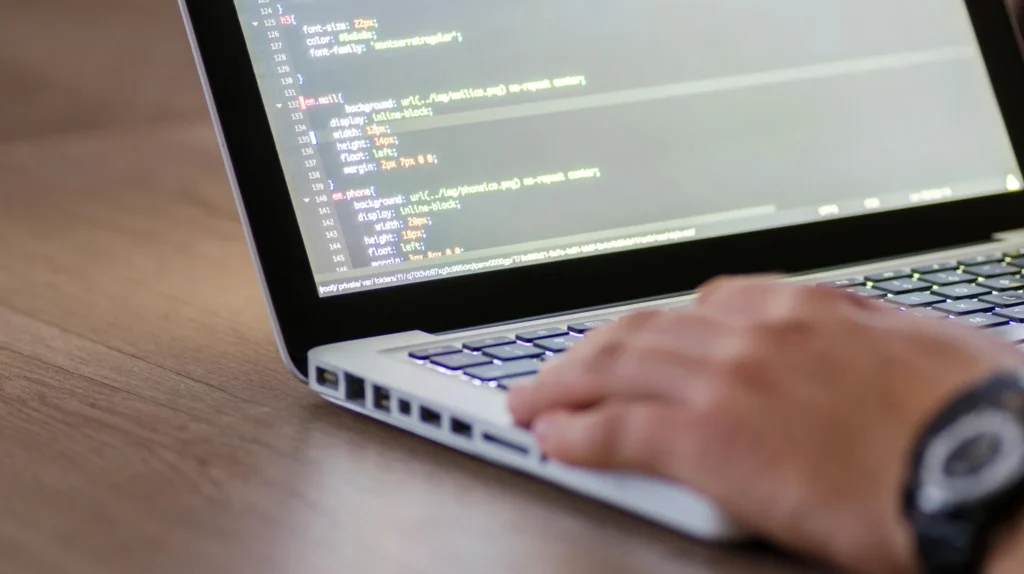
Featured Insights
Logging Exceptions in Salesforce
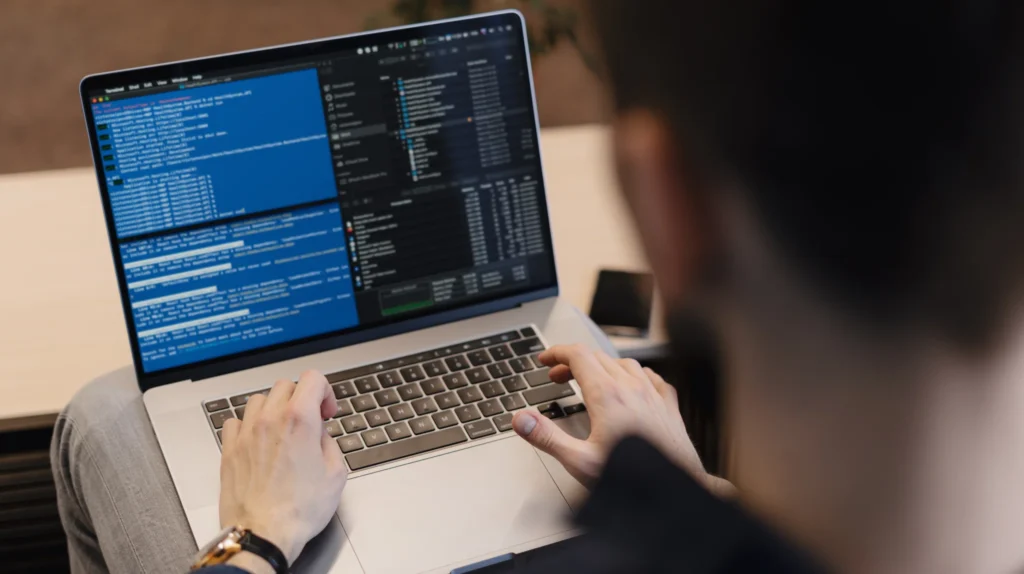
Featured Insights
New Intranet Solutions In The Microsoft 365 Ecosystem
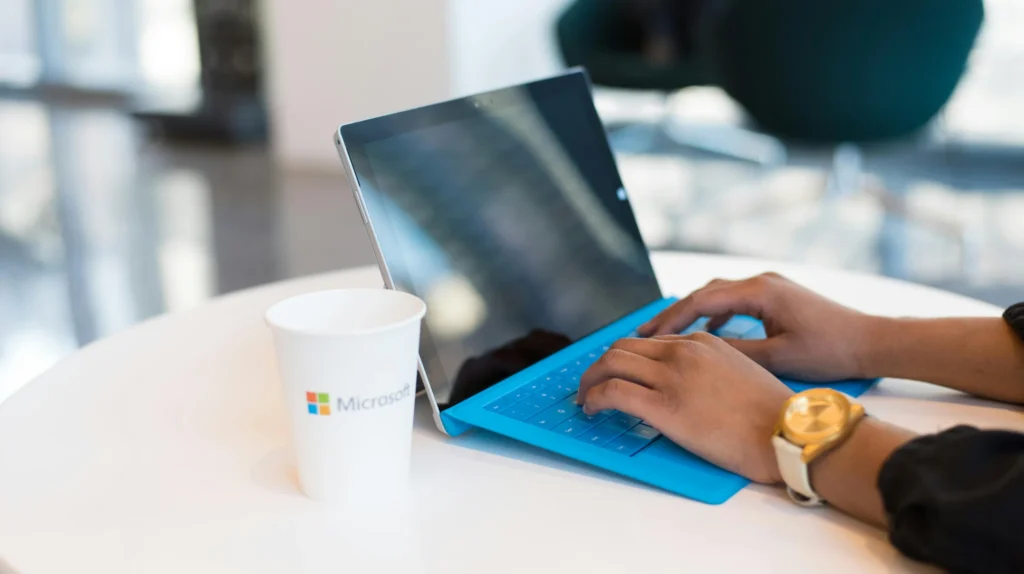